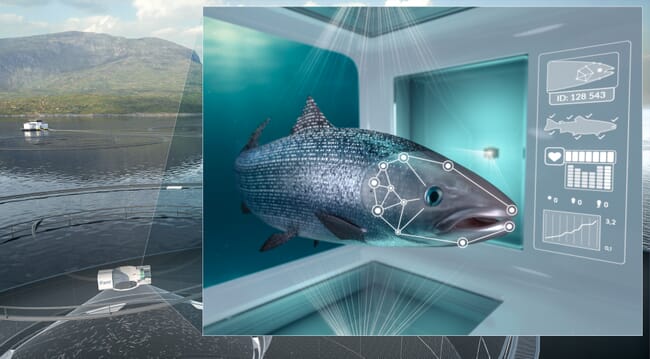
Aquaculture has been slowly embracing artificial intelligence (AI) to assist in husbandry and other production tasks © Cermaq
Several prominent news stories have circulated over the past few months describing advances in the application of AI in our industry. Of course, for those of us with limited experience in the field of advanced computer programming, one of the first questions that arise is usually “just what exactly is AI”?
Without going into too much detail, AI involves programming that facilitates recognition (images, languages, music, etc.) and decision making – without the need for human supervision. Oxford Languages defines AI as “the theory and development of computer systems able to perform tasks that normally require human intelligence, such as visual perception, speech recognition, decision-making, and translation between languages”.
How did we get here?
The history of AI as it is currently being applied to aquaculture began with something called Fuzzy Logic. In 1965, Lotfi A Zadeh at UC Berkeley published an academic article titled “Fuzzy Sets”. He presented the novel concept of classes of objects displaying “a continuum of grades of membership”. This concept emphasised that many things do not fall clearly into one category or another, and set the stage for what is now known as fuzzy logic.
While traditional computing of the time utilised values of 0 or 1 and true or false, the theory behind fuzzy logic embraced the reality of human perception, where things are often somewhere between 0 and 1 or true and false. Over the years, fuzzy logic has lent itself to computer applications like image processing and disease diagnosis, and it has been incorporated in control systems for everything from automotive transmissions to dishwashers to photocopiers.
I was first exposed to the possibility of applying artificial intelligence in aquaculture more than two decades ago. Lee et al. (2000) at the University of Texas Medical Branch in Galveston published a summary of their use of a fuzzy logic-based control system for denitrification in a closed recirculating system. Their work focused on development of a computer-controlled denitrifying bioreactor for a system housing squid for biomedical research. Fuzzy logic was used to process real-time inputs from sensors measuring dissolved oxygen, oxidation-reduction potential and pH, and in turn to control pumping rates and carbon feed additions to the bioreactor.
AI technology has developed rapidly over the past two decades in many fields, often in ways that can easily be adapted to aquaculture production. Two advances that have particular impacts are deep learning and convolutional neural networks. Traditional machine learning uses algorithms to perform functions with the data being supplied, but in a way that becomes more efficient over time through feedback and adjustments (partially from human input). Deep learning goes one step farther by layering algorithms and self-evaluation and adjustment. Deep learning systems learn through their own computing processes. Convolutional neural networks are deep learning algorithms that are particularly useful for image recognition and interpretation.
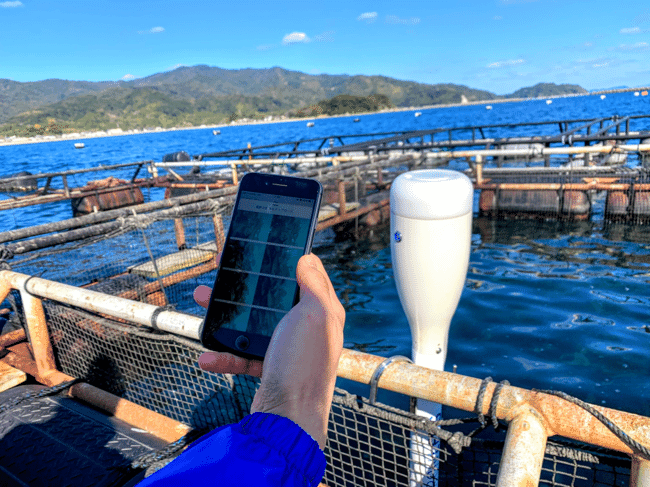
AI is currently being evaluated and deployed in aquaculture for improving feeding efficiency, biomass estimation, growth tracking, early detection of diseases, environmental monitoring and control (especially in RAS) and reduction of labour costs © Umitron
At the same time, sensor technology has advanced by leaps and bounds, as have connectivity options through the Cloud, 5G networks and the internet of things (IoT). As a result, AI is currently being evaluated and deployed in aquaculture for improving feeding efficiency, biomass estimation, growth tracking, early detection of diseases, environmental monitoring and control (especially in RAS) and reduction of labour costs. With modern sensor and processing technologies, many of the routine tasks of modern aquaculture can be performed with much less labour and improved animal welfare conditions.
Current research
Advanced disease detection based on fish behaviour and external appearance has emerged as a promising area for AI application. Chen et al. (2022) reported on a two-phase image analysis system using deep learning and a convolutional neural network to classify three types of abnormal appearance in cage-cultured grouper. Of four classification models developed in the study, the best achieved an average accuracy of 98.94 percent.
Costs of components required to elaborate AI systems for fish farming are still relatively high, but cost trends and novel approaches may eventually extend accessibility to even the most resource-limited producers. Recently, Darapaneni et al. (2022) proposed a system specifically for early detection of disease outbreaks, providing artisanal farmers with more time to respond with suitable management options. The system relies on underwater cameras or similar sensors to obtain images that are passed on, via the Cloud, to a partner for processing and scoring. Subsequently, the data will be classified and analysed via a trained AI model. With modern connectivity options, turnaround time can be as little as a matter of minutes and several or more farms can be evaluated per day.
The Canadian firm ReelData AI has developed two products for land-based aquaculture operations © Reel Data AI
More efficient feeding practices have also been the focus of recent aquaculture AI research. Chen et al. (2021) used a biomass prediction formula based on a support vector machine model using real-time water quality data in conjunction with artificial neural networks to predict feed requirements and optimum feeding amounts for shrimp reared in an RAS facility. The results indicated a mean percentage error of 3.7 percent, probably far better than manual feeding could achieve.
Biomass monitoring and inventory control are also fertile areas for AI applications in aquaculture. Gonçalves et al. (2022) described the use of a convolutional neural network for counting fingerlings. The AI approach involved sequential images that allowed for enumeration of fish even when two or more individuals were in contact or overlapping each other, and the model was adaptable to predict fingerling movements. Overall, F-measures (the harmonic means of precision and recall, with 1.0 being a perfect score) reached 97.89 when temporal information was included in the model. Natsuike et al. (2022) used a deep learning technique known as “semantic segmentation” in conjunction with approximately 9,000 time-lapse images to identify individual scallops in lantern nets and track their growth and behaviour patterns.
Some real world applications
While some industry observers and stakeholders may be unsure about the future of AI in production aquaculture, or hesitant to embrace it wholeheartedly, others are taking the plunge in a big way. The Canadian firm ReelData AI has developed two notable products for land-based aquaculture operations: ReelAppetite monitors and adjusts feed consumption and provision, while ReelBiomass provides estimates of size and weight distributions within production facilities. The company recently made headlines as a result of raising over $8 million USD in its series A funding round.
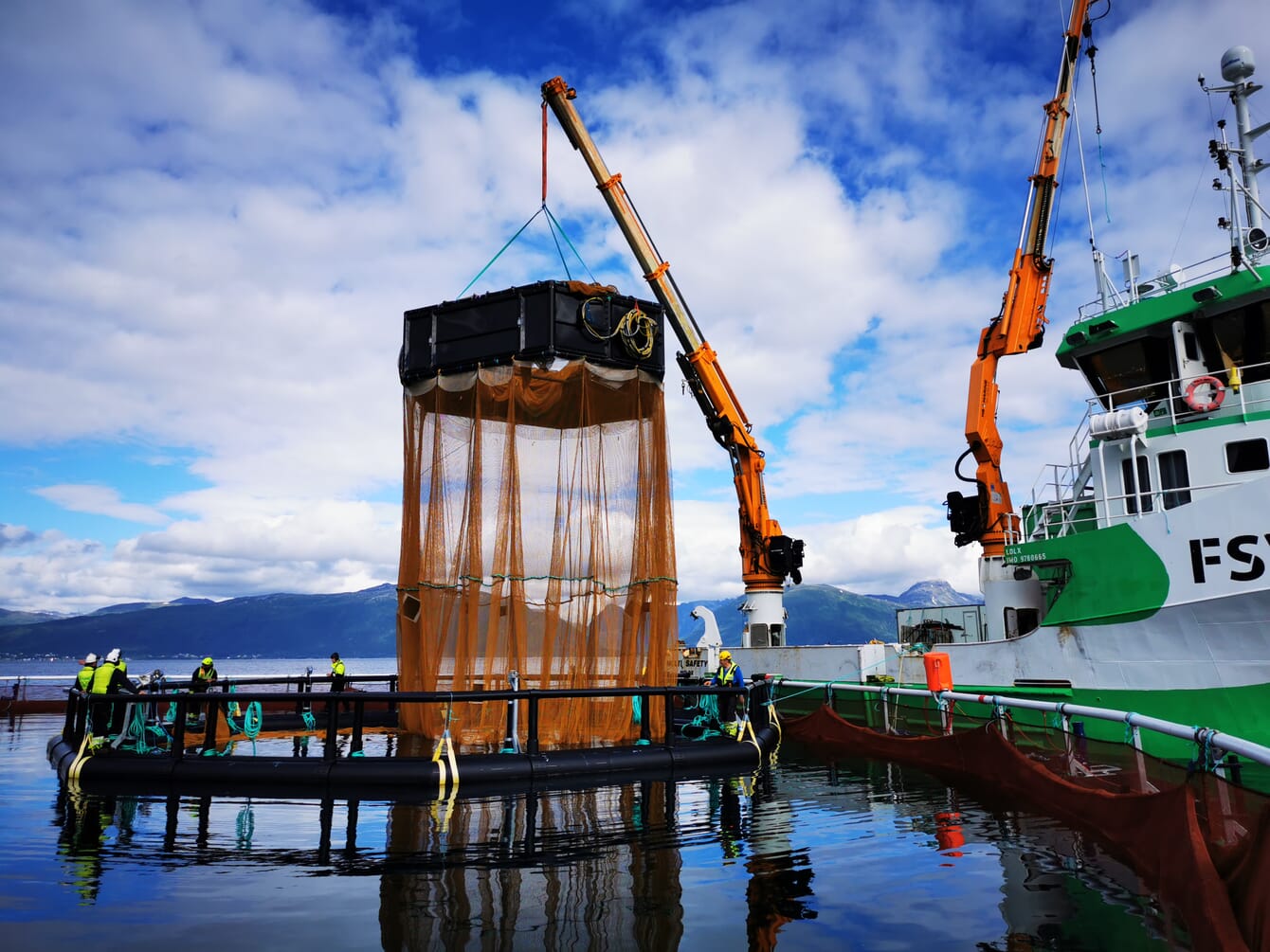
The iFarm concept was developed by the technology partner BioSort to improve fish health and welfare in net pens © Cermaq
Tokyo-based Umitron Corporation has been focusing its efforts on adapting AI technology for aquaculture applications. The Kura Sushi restaurant chain has been farming mackerel tuna at its Kura Osakana production site, using AI systems developed by Umitron. Umitron’s system utilises real-time monitoring of swimming behaviour to make decisions regarding when and how much feed to dispense to each fish cage. This approach improves feed conversion efficiency significantly while reducing wastes, and greatly reduces transport/logistics requirements associated with conventional daily feeding. The fish are now being marketed as “AI Sumagatsuo.”
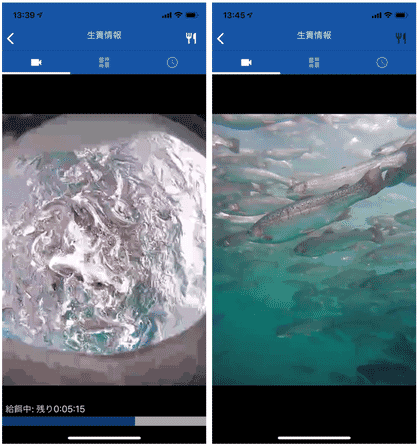
Umitron’s system utilises real-time monitoring of swimming behaviour to make decisions regarding when and how much feed to dispense to each fish cage © Umitron
The global salmon producer Cermaq has also been working for several years now with an AI system. Dubbed iFarm, the concept was developed by the technology partner BioSort, with the overall goal to improve fish health and welfare in net pens. The research has involved incremental goals. Initially, the company focused on how the fish interacted with the system, and subsequent trials aimed to adapt and streamline system components and operations. Current trials are evaluating sensor technologies, data acquisition and processing and machine learning algorithms. Developing methods to sort fish within net pens has also become a priority, with the goal of monitoring and treating individual fish, based on their unique dot patterns and the presence of sea lice.
The next steps
Technology is advancing rapidly in every aspect of AI-based monitoring and control for aquaculture. Modern devices can now allow for the use of stereoscopic observation of the size, shape, position and behaviour of fish and shrimp. “Sonar cameras” can now convert sound echoes to video images for use in dark or turbid environments. Water quality monitoring can be done in three dimensions in cages and large tanks by using autonomous vehicles that raise and lower sensors to generate 3-D data profiles. While indoor (RAS) and underwater (net-pen) production environments are comparatively stable, more environmental challenges may be encountered for equipment deployed in open ponds in the future.
Wu et al. (2022) provided a broad overview of the current and potential uses of AI in aquaculture. They outlined the technological evolution of many devices from manual activities through mechanisation, automation and – ultimately – intelligent equipment. One important observation made by these authors is the potential for improved biosecurity through the use of unmanned equipment, and the development of autonomous robots is rapidly expanding in the net-pen farming sector. These units have great potential for maintaining sanitary conditions by collecting and removing mortalities, uneaten feed, and accumulated waste, as well as for net cleaning. Costs are still somewhat prohibitive for some operations but are expected to come down in the coming years.
Current iFarm trials are evaluating sensor technologies, data acquisition and processing and machine learning algorithms
Mustapha et al. (2021) reviewed many of the topics presented in this article, and also pointed out a number of constraints and challenges that may complicate the widespread adoption of AI in aquaculture. Among these are security concerns related to the many levels of the interconnected systems and networks that will be required. Another issue will be the need for complex data acquisition to meaningfully capture the complexity and biological interactions found in many production systems. Deep learning AI requires large amounts of training data, which is typically difficult to come by. For some applications, this is further compounded by the turbidity and bio-fouling associated with many culture systems. Finally, cost-benefit considerations will largely determine the extent and pace of AI adoption. But the long term trends for adoption are increasingly apparent.