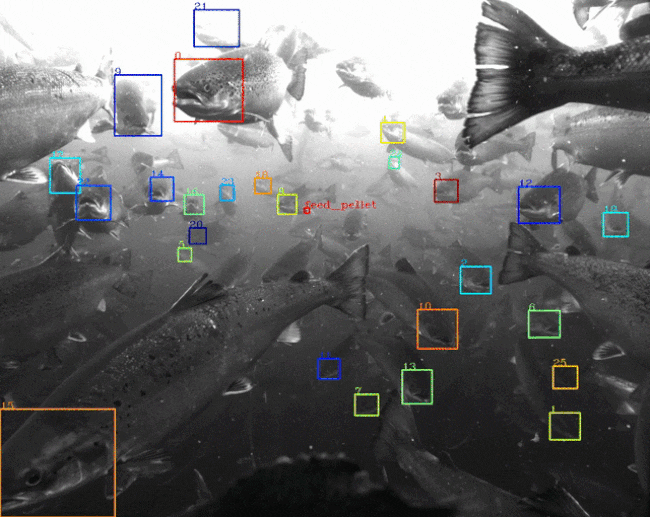
© Tidal
Parts of the aquaculture industry – in particular the salmon sector – are experiencing an unprecedented technological revolution, with the development of AI-based devices for counting and inspecting fish, autonomous underwater robots, sea lice lasers, and a variety of IoT sensors and decision-making tools.
This represents a fundamental reimagining of how we farm seafood, and a growing number of companies are developing AI-based tools and technologies tailored explicitly for aquaculture. However, with this influx comes the challenge of discerning genuinely useful AI applications from those simply riding the AI wave, leaving clients struggling with implementation and value realisation.
AI 101: Understanding the fundamentals
AI is an umbrella term encompassing various technologies that enable machines to mimic and enhance human intelligence. Within this field, several concepts are critical to understand:
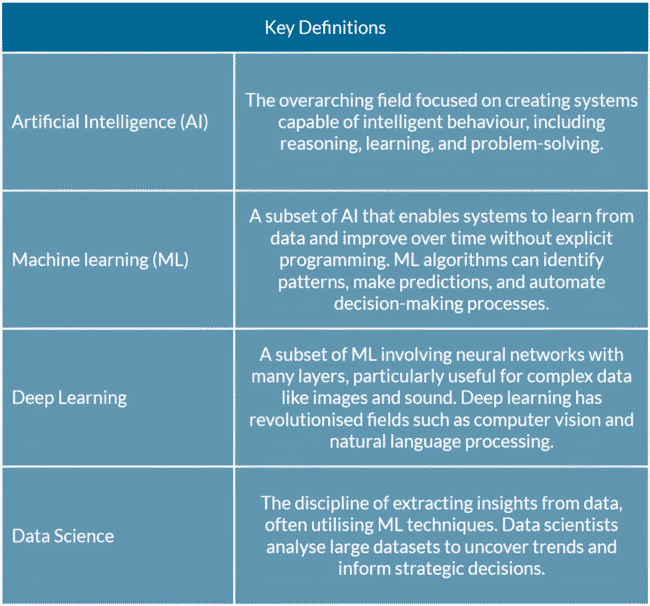
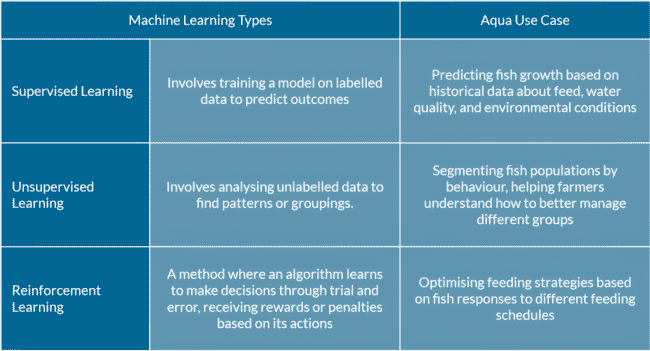
Understanding these fundamental concepts is essential for aquaculture professionals aiming to implement AI tools effectively. By grasping the various types of machine learning and their applications, we can better assess how AI can add value to their operations.
The AI wave in aquaculture
AI tools are integral to the evolution of the aquaculture industry, providing data-driven decision-making for more informed management strategies. Some – primarily focusing on feeding optimisation, biomass estimation and fish behaviour – are already quite mainstream in the farming of high value species.
AI-driven systems, such as those developed by Skretting, AKVA Group, and Innovasea, monitor fish behaviour in real time to dispense the exact amount of feed needed, significantly reducing waste and improving growth rates. These systems adjust feeding schedules and formulations, based on data related to fish growth and environmental factors, aiming to enhance feed conversion ratios and substantial cost savings, while minimising environmental impact.
Others, such as those offered by Tidal X, Umitron, ReelData and Optoscale, use AI-powered cameras and algorithms to provide real-time data on fish sizes and growth projections, aiming to improve operational efficiency and resource planning.
Companies like Manolin* and Aquabyte use big data and AI to aggregate health and welfare-related information. This allows early disease detection and timely responses to potential outbreaks. Other companies like Qysea are incorporating AI algorithms within underwater drones to perform fish and water quality monitoring, net repairs and cleaning, and dead fish removal.
Research institutions and universities are also working on various solutions (independently or in collaboration with industry players). These include the ShrimpWiz project, led by the Alfred Wegener Institute in cooperation with Oceanloop, for individual shrimp counting; the University of Aberdeen & SAMS (supported by Mowi) for microscopic sea lice larvae detection; SoftBank and Aizip’s collaboration on a fish counting mobile app with an AI question-answering programme.
Emerging sector landscape analysis
According to Hatch Blue’s database, as of February, 125 startups are developing solutions involving AI for aquaculture, with North American and European-based companies leading the way.
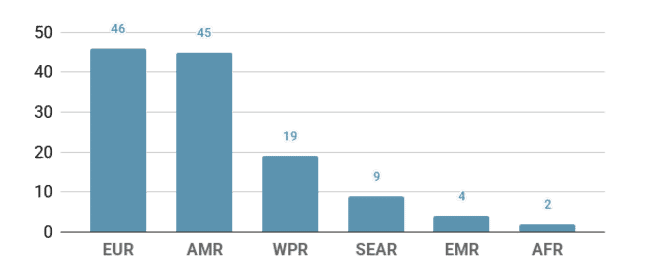
WPR = Western Pacific Region; SEAR = South East Asia Region; EMR = Eastern Mediterranean Region © Source: Hatch Blue Consulting
Around 90 percent of these are still at an early stage, focusing on product development, market validation and initial customer acquisition. This suggests the high interest and market opportunities within the sector but also indicates that a relatively low number of companies have successfully navigated the earlier phases and are now focused on scaling their operations, expanding their market reach, and increasing revenues.
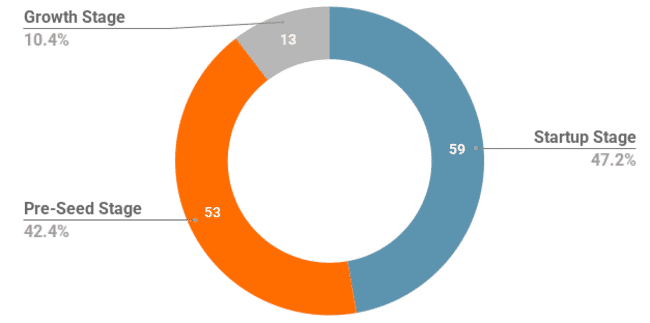
© Hatch Blue Consulting
Several initiatives also target downstream applications to streamline the aquaculture supply chain from farm to market. Aquaconnect* uses AI and satellite remote sensing to connect farmers and seafood buyers, aiming to bring predictability and transparency to the aquaculture supply chain.
Additionally, The Fish Site recently launched Seashell AI, the first dedicated AI assistant for aquaculture, which uses large language model (LLM) capacities on validated aquaculture databases to optimise research and learning in the field.
Meanwhile, the US FDA has launched a pilot programme, focusing on the imports that make up over 90 percent of the country’s seafood supply. It aims to enhance food safety by using ML to identify potential hazards in the supply chain. The third phase of the pilot, started in August 2022, focuses on quickly detecting contaminated seafood and other risks, such as unapproved antibiotic residues.
Apart from core software and hardware companies, most AI-related companies in our database are targeting supply chain and services, and next generation farming applications.
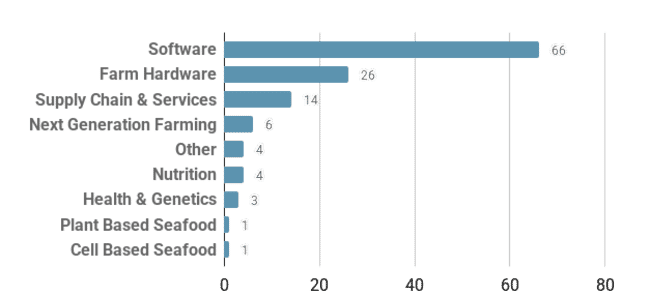
© Hatch Blue Consulting
A reality check
While the potential benefits of using AI-powered tools are widely promoted, some challenges can be overlooked:
- High implementation costs: The initial investment in AI technology can be prohibitive, especially for small and medium-sized businesses. Sometimes, a simple and affordable oxygen sensor with an alarm system can be more effective than sophisticated and complex algorithms and IoT sensors trying to detect abnormal fish behaviour.
- Technical expertise requirements: Many farmers lack the technical knowledge to implement and maintain AI systems effectively.
- Data quality and quantity: Deep learning requires large volumes of high-quality training data, which can be challenging to obtain in turbid aquaculture environments.
- Security concerns: Interconnected systems create potential vulnerabilities in data security and system integrity.
- Uneven global distribution: AI adoption in aquaculture is concentrated in developed regions, with limited penetration in Africa, Latin America, and small island developing states.
As AI gains popularity, some companies may overstate their use of AI or falsely claim to have solutions that are not yet developed or do not align with the technology's true capabilities. This can create confusion or mistrust about what AI can genuinely achieve. It's crucial for businesses to investigate these claims and focus on tangible benefits rather than marketing buzzwords. Customers can waste a lot of time and money by not fully understanding the right solution for their needs and what internal stages their data needs to go through to be “AI ready”. Additionally, businesses may find it challenging to justify the investment required to achieve tangible results. Consequently, education and expert guidance are essential to distinguish the value of available solutions and what is required for an effective implementation or integration.
To evaluate AI claims in aquaculture, we can suggest several high-level guidelines:
- Ask for specific metrics: Request concrete performance data comparing AI systems to traditional methods.
- Understand the technology: Legitimate AI providers should be able to explain how their systems work without resorting to vague terminology.
- Check references: Speak with existing customers about their experiences with the technology.
- Start small: Test AI solutions in limited applications before committing to full-scale implementation.
- Consider the business model: Be cautious of companies focusing more on raising venture capital than developing practical solutions for farmers.
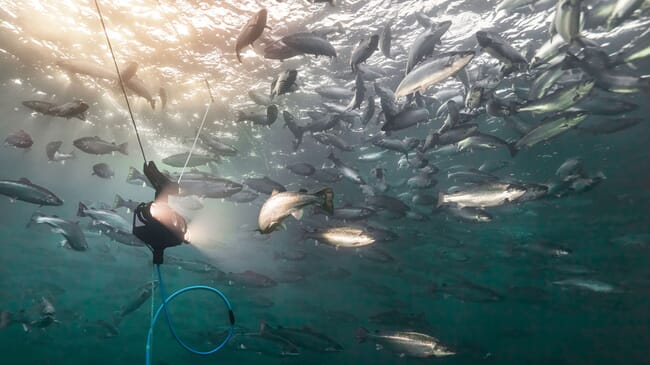
© Aquabyte
Preparing for AI Integration
To maximise the benefits of AI in aquaculture, it's crucial to ensure data quality, invest in robust infrastructure, and prioritise cybersecurity measures. For aquaculture businesses aiming to adopt AI, preparing their internal databases is a crucial first step. This preparation is not merely a technical upgrade; it requires a comprehensive approach to data management.
While large operations generate substantial data from various sources such as sensors and cameras, this data is often fragmented and inconsistent, lacking the necessary labelling for supervised learning applications. Smaller aquaculture operations may struggle to consolidate and manage this data effectively due to limited infrastructure and expertise, making the data-cleaning process both time-consuming and resource-intensive. Additionally, data privacy and security concerns complicate matters, as sensitive information must be protected when shared with third-party providers or cloud platforms, raising the stakes for robust governance policies and encryption measures.
Integration with existing operational systems further complicates the process. Many facilities utilise legacy systems not designed for AI, necessitating significant engineering efforts to ensure compatibility, which may disrupt ongoing operations. Moreover, the aquaculture industry often lacks skilled data science and machine learning professionals, making developing and deploying in-house AI solutions challenging. Finally, successful adoption hinges on effective change management, as employees may resist new technologies without proper training and support.
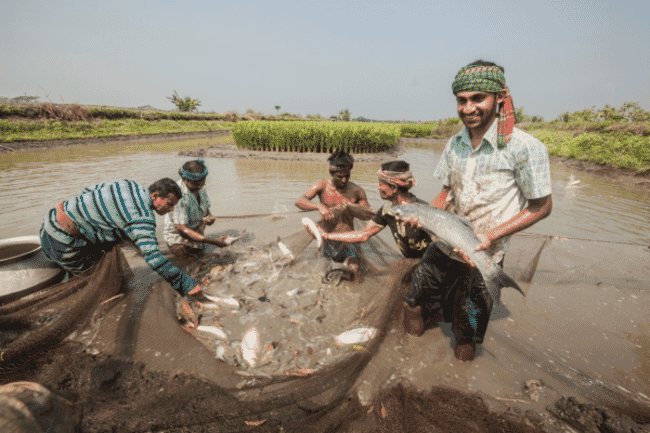
© Aquabyte
Introducing the HI factor
Despite the excitement surrounding AI, we should still recognise the irreplaceable value of human intelligence (HI), the ability to interpret complex, unpredictable situations with experience, ethical judgment, and adaptability – elements that AI currently lacks. HI is particularly relevant in aquaculture, where success depends on understanding complex biological and chemical systems and making nuanced decisions based on multiple factors. Aquaculture experts bring contextual understanding, intuition, and adaptability that AI systems – at least current ones – cannot replicate.
Humans provide essential contextual understanding, enabling experienced professionals to interpret data anomalies effectively and make strategic decisions considering external factors like the latest market trends and moral concerns. Furthermore, in crisis situations, such as disease outbreaks or environmental changes, human intuition and problem-solving skills are vital for navigating complexities that AI alone cannot address. While AI can optimise operations, human oversight can ensure sustainability and virtuous practices.
The way forward: balancing technology and tradition
As AI continues to evolve in aquaculture, the most successful implementations will likely be those that strike a balance between technological innovation and traditional knowledge. It's essential to recognise that the wisdom and experience of experts can often inform practices just as effectively, if not more so, than algorithm-driven solutions. Using AI is not just about enhancing productivity; it’s about creating a sustainable future for aquaculture that balances technological advancement with ethical and ecological considerations.
*Manolin and Aquaconnect are part of Hatch's investment portfolio, but The Fish Site retains editorial independence.